Recently, the research group led by Professor Fang Lu from the Department of Electronic Engineering at Tsinghua University, and the research group led by Professor Dai Qionghai from the Department of Automation, innovatively designed a fully forward intelligent optical computing training architecture and developed a general optical training chip named "Taiji-II".
This architecture has broken away from the dependency on offline training with electronic computing, and can provide support for efficient optical training of intelligent systems.
The research team established a photon propagation symmetry model, did not adopt the traditional electronic training backpropagation paradigm, turning the "back" to "forward".
This new paradigm has freed itself from the strict alignment of forward-backward optical field propagation, based on a universal optical computing in-situ training system, breaking through the constraints of electronic training architecture on physical optical computing.
The research provides the field of optical computing with a precise and efficient training method for large-scale neural networks, provides new ideas for the design and development of intelligent optical computing systems, and opens up new boundaries for the computing power of light.The reviewer of the study commented: "The ideas proposed in this paper are very innovative, and the training process of such optical neural networks is unprecedented. The method proposed by the authors is not only effective but also easy to implement. Therefore, it is expected to become a widely adopted tool for training optical neural networks and other optical computing systems."
Advertisement
Recently, the related paper was published in Nature under the title "Fully forward mode training for optical neural networks" [1].
Tsinghua University doctoral student Xue Zhiwei and postdoctoral fellow Zhou Tiankuang are the co-first authors, Professor Fang Lu and Professor Dai Qionghai serve as co-corresponding authors. Tsinghua University doctoral student Xu Zhihao and Dr. Yu Shaoliang from Zhijiang Laboratory participated in this research.
Unleashing the "training power" of intelligent optical computing.In recent years, with the rapid development and widespread application of AI large models such as ChatGPT, Sora, and Llama, there has been an increasing demand for computational power.
Training a typical neural network requires hundreds of thousands to tens of millions of parameters, while the parameter volume of large models can reach several billion.
Electronic computing chips have always supported the continuous development of model scales, but an inescapable issue is that high computational power under electronic computing architecture also means high energy consumption.
Taking the training of GPT-3 as an example, according to the "2023 Artificial Intelligence Index Report" released by the Stanford Institute for Artificial Intelligence in the United States, the power consumption required for training a large model once is 1287 megawatts.
Therefore, at this stage, the development of AI not only needs to solve technical and computational problems but also means facing energy issues. The previous paradigm can no longer effectively solve the current problems, so the development of emerging intelligent computing paradigms has emerged.Light possesses multi-dimensional computational modalities such as interference and diffraction. Using light as a computational carrier, we can construct computational models with the controllable propagation of light. Compared to electronic computation, under the premise of completing the same computation, optical computation can achieve related neural networks with faster speed and lower energy consumption.
This implies that optical computation, with its advantages of high computational power and low energy consumption, serves as a "dark horse" in intelligent computation, bringing new hope to the post-Moore era.
Inference and training are two important stages of the core capabilities of AI large models. Recognizing this, the team conducted concurrent parallel research in inference and training.
In April of this year, they reported on the universal intelligent optical computing chip "Tai Chi" in Science [2], for the first time promoting optical computation from principle verification to large-scale experimental application. Its system-level energy efficiency is 160TOPS/W, allowing people to see more possibilities for the inference of complex intelligent tasks.
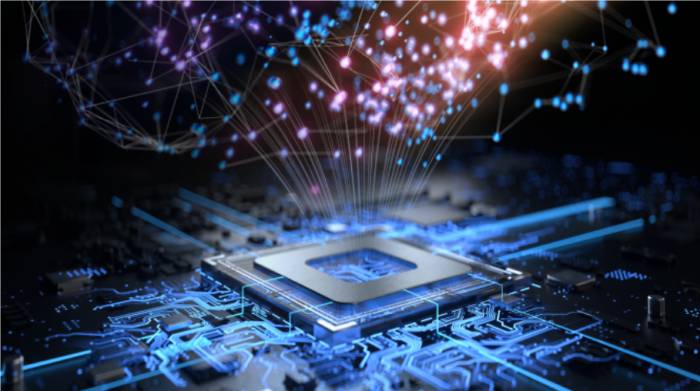
However, the initial research on the first generation "Tai Chi" (Tai Chi-I) focused on inference and has not yet released the "training power" of intelligent optical computation. The focus of the Tai Chi-II in this study is on training.Break free from the reliance on offline training with electronic computation, and surpass the current scale limits of optical networks that support training.
Compared to model inference, the demand for computational power is more urgent for model training. However, the training of existing optical neural networks heavily relies on offline modeling and optimization through electronic computation.
The electronic training architecture requires a high degree of alignment with the forward-backward propagation model, thus setting higher demands for the precise alignment of the optical computing physical system. This often leads to challenging gradient calculations, slow offline modeling, and large mapping errors, greatly limiting the scale and efficiency of optical training.
To address the aforementioned issues, researchers have proposed a solution that is reciprocal and optically common.
- Reciprocal: A fully forward intelligent optical computing training architecture.Inspired by the symmetry of physics, researchers have established a dual-symmetric optical propagation model of "spatial reciprocity-time reversal," and proposed a fully forward intelligent optical computing online training architecture.
Xue Zhiwei explained, "Transforming the backpropagation in gradient descent into the forward propagation of the optical system, the two forward propagations actually follow exactly the same path, thus possessing a naturally aligned characteristic, which ensures the accuracy of the physical gradient calculation."
This architecture breaks through the dependence on offline modeling and training of electronic computing, equivalently mapping neural network training to the forward propagation of light. Its high-speed and low-power characteristics greatly enhance the speed and energy efficiency of training, laying the foundation for supporting large-scale network training.
ยท Optical commonality: Universal intelligent optical training empowers complex systems.
Starting from the basic principles of wave optics, the team proposed a universal differentiable neural representation of multi-scale optical systems, using modulation and propagation to construct any optical system.Researchers have established a mapping relationship between the modulation and propagation of physical optical systems and the activation and connection of neural networks, meaning that the training of the modulation module can drive the weight optimization of any network, thereby ensuring the speed and energy efficiency of training.
Xue Zhiwei said: "Through this new type of optical system, we provide a 'light-speed' solution for the online training of complex physical systems."
The actual measurement results of the system show that the Tai Chi-II intelligent optical training architecture has demonstrated excellent performance in large-scale learning, intelligent imaging in complex scenes, and topological photonics.
Specifically:
In the field of large-scale learning, it provides a solution for the 'not easy to obtain' computational accuracy and efficiency. Compared with the training speed of the previous optical network with millions of parameters, the optical training speed of Tai Chi-II is 1 order of magnitude faster, while the accuracy of representative intelligent classification tasks is 40% higher.In the field of intelligent imaging in complex scenes, the intelligent imaging effect reaching a frame rate of kilohertz has improved the imaging efficiency by two orders of magnitude.
In the field of topological photonics, Tai Chi-II can automatically search for non-Hermitian singularities without relying on prior models, making it possible to efficiently and accurately analyze complex topological systems.
The research began at the end of 2021. Initially, they completed the linear network, but when they advanced to the nonlinear large-scale network, they encountered significant challenges.
Although the two are theoretically relatively consistent, it is not easy to complete the nonlinear network in practice. Sometimes, the team members have no experimental progress for several weeks in a row.Currently, Xue Zhiwei is in his third year of pursuing a Ph.D. in the Department of Electronics at Tsinghua University. It is reported that this is his first work during his doctoral period, which took a full 3 years of careful polishing before finally bearing fruit.
Recalling the experimental process of the research, Xue Zhiwei said, "On a winter morning in Beijing, after a long period of optimization and debugging, the system that had not been working well before finally started to work, which means that the experiment has approached the theory. I remember when I walked out of the laboratory, I felt that the wind was sweet."
It is expected to provide computational support for AI large models in the post-Moore era.
Inspired by the physical characteristics of optics, Tai Chi-II proposes a technical path that is not based on electrical training architecture.
Using a fully forward optical propagation model to solve the problem of large-scale network training, it overcomes the bottlenecks of poor computational accuracy, slow training speed, and low energy efficiency, thereby supporting efficient and high-precision online training of multi-scale complex optical systems.It is understood that the current research team has successfully completed the preparation of the prototype and is advancing towards the industrialization of intelligent optical chips, deploying applications in end-side intelligent systems including drones, autonomous vehicles, and robots.
The transformation of technology from academia to industry is a complex process. Although the Tai Chi-II chip itself has extremely low power consumption, there are still related challenges in the engineering process of on-chip light sources, on-chip optical storage, and peripheral electronic equipment.
To further explore and develop these technologies, the research team is actively promoting close cooperation with the industry and research institutions to promote further integration and optimization of optical chip systems.
"We hope to achieve a product-level optical computing system with complete packaging of optical chips and peripheral equipment within 2-3 years, gradually realizing the transformation of chips from dedicated applications to general applications," said Xue Zhiwei.
The introduction of Tai Chi-II brings new dawn for intelligent optical computing in large-scale training. It will work together with Tai Chi-I to create a new base for optical computing power, providing a new solution for the development of computing power for AI large model training and inference."Tai Chi" is not only a series of intelligent photonic computing chips, but also a dialectical collaborative architecture that possesses the unique dualistic attributes of photonic computing systems. Just as their names suggest, Tai Chi I and II are both separate parts and a unified whole when combined.
The team, through the related research of Tai Chi-I and Tai Chi-II, has completed the exploration of AI inference and training, and together they constitute the entire lifecycle of large-scale intelligent computing, ushering in a new era of intelligent photonic computing.
The completion of this research allows people to see the relentless voyage of the intelligent photonic computing power ship, including the Tai Chi series of photonic chips. The intelligent photonic computing platform is ultimately aimed at solving the problems faced by AI computing power, and will sail towards the vast sea of AI computing power, providing a new solution for green and efficient AI large models, general artificial intelligence, and so on.